Precision agriculture offers the possibility of balancing maximum productivity with conserving and protecting the land for future generations, but the challenge has been finding capable tools that can make both goals achievable...
January 6, 2023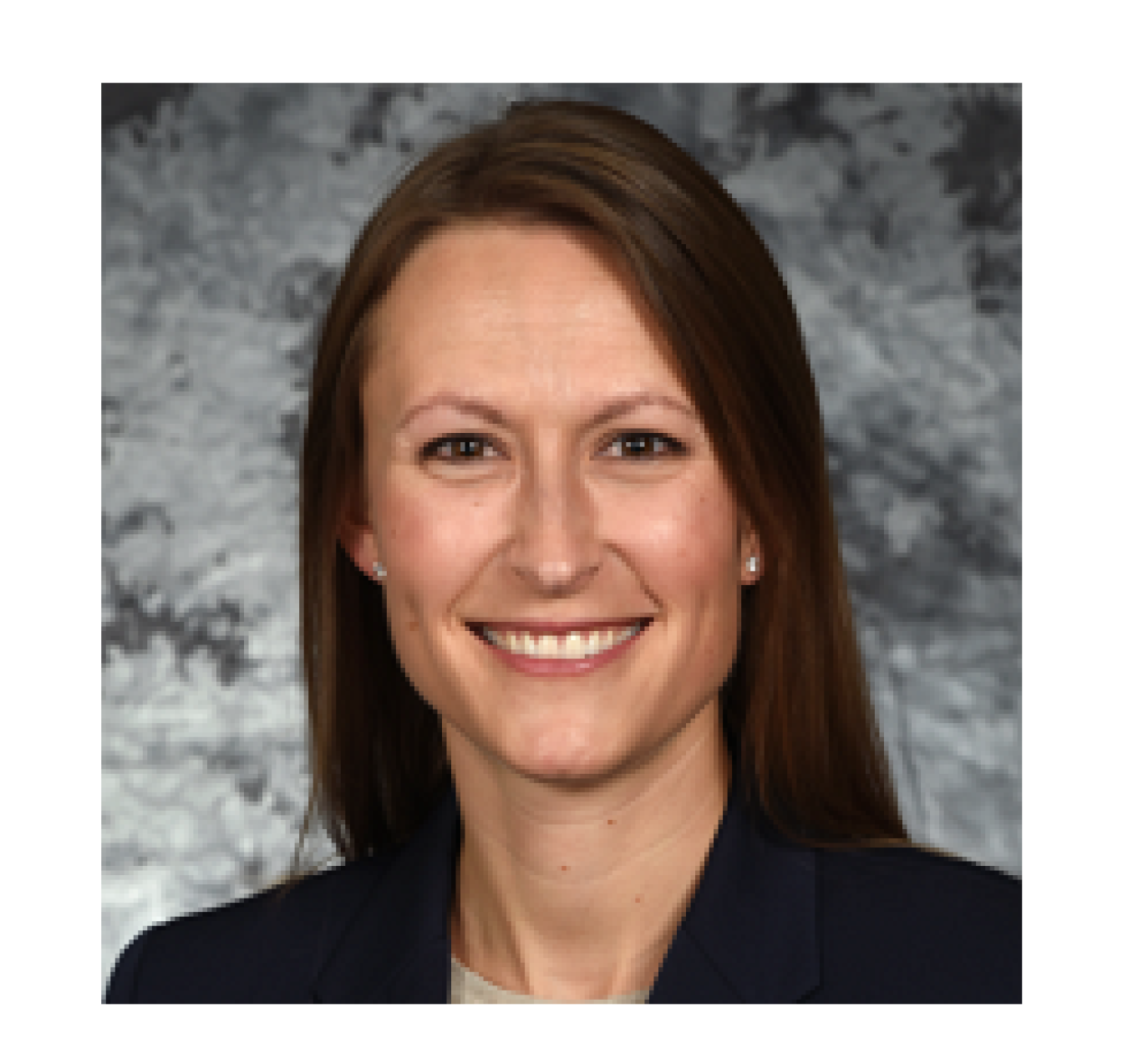
Author: Dr. Lynn Wendt, Laboratory Relationship Manager, Idaho National Laboratory
Read Lynn's bio ►
Meet the other bloggers ►
Return to Bioprose blog ►
Precision agriculture offers the possibility of balancing maximum productivity with conserving and protecting the land for future generations, but the challenge has been finding capable tools that can make both goals achievable. Idaho National Laboratory (INL) researchers recently discovered a way to use artificial intelligence (AI) to do just that.
Many farms have land where, because of poor soil conditions or inadequate drainage, row crops like grain, corn, and soybeans cannot be grown profitably, even using precision agriculture. That land can still be productive, though, since energy crops like switchgrass and miscanthus are often well-suited to poorer-quality ground.
Accurate, up-to-date yield maps analyzing variables like crop yield and moisture can help growers apply precision treatments (e.g., pesticides) only where needed, saving time and money while simultaneously benefitting the environment. Through support from the U.S. Department of Energy (DOE) Bioenergy Technologies Office (BETO), INL researchers transformed traditional yield mapping into a more efficient and sustainable tool for farmers.
INL Advances Yield Mapping for the 21st Century
With all the technological advances in farm equipment and software, yield mapping has remained a time-consuming and outdated relic of the past. Crop Artificial Intelligence Quotient (Crop AIQ), a computational tool that supplies yield data quickly in an easy-to-understand format can change that. A 2020 R&D 100 Award winner, Crop AIQ uses publicly available satellite imagery data sets to render yield maps at high subfield spatial resolutions. In bushels per acre, Crop AIQ enables growers to estimate expected crop yields in subfields, making adjustments based on which specific crops may be most profitable.
“Farmers like to drive in a straight line for as long as possible,” said Mike Griffel, INL’s principal investigator for Crop AIQ. “It’s a challenge for farmers to change their crop rotations. But if redesigning a field will optimize the economic outcome, a farmer is likely to be more receptive to switching to a different crop they aren’t used to."

Figure 1. Map shows the yield potential of farming land. Image courtesy of INL
The Nuts and Bolts of Crop AIQ
Crop AIQ uses an input of U.S. Department of Agriculture satellite-based remote sensing data that spans the visible, near infrared, and shortwave infrared (SWIR) electromagnetic spectrums. This remote sensing measures the reflectance intensity across the electromagnetic spectrum. These intensities change during the growing season due to shifts in crop canopy structure, photosynthetic activity, and chlorophyll production. The changes provide a feedback signal that can be measured and interpreted to produce a predicted yield outcome. Crop AIQ was developed to use publicly available open-source spectral datasets consisting of 12 bands that run from 490 nanometers in the blue light range to 2,190 nanometers in the SWIR spectrum.
The model was trained with information from yield monitors on harvesters working in multiple fields of corn, wheat, and soybeans in Idaho and Minnesota from 2016 to 2018. Using error-correcting feedback and working on a training set of fields, the artificial neural network adjusted the weight given to various features within the spectral data so that its predicted yield closely matched the actual yield. The model developers then used the resulting software to predict the yield from other nearby fields that were not in the training set. This check proved the validity of the model.
There are many options for industry deployment of Crop AIQ. Ideally, the model framework could be housed in a web interface where a farmer could add field boundaries and generate a yield map based on specific crop type and available remote sensing data.
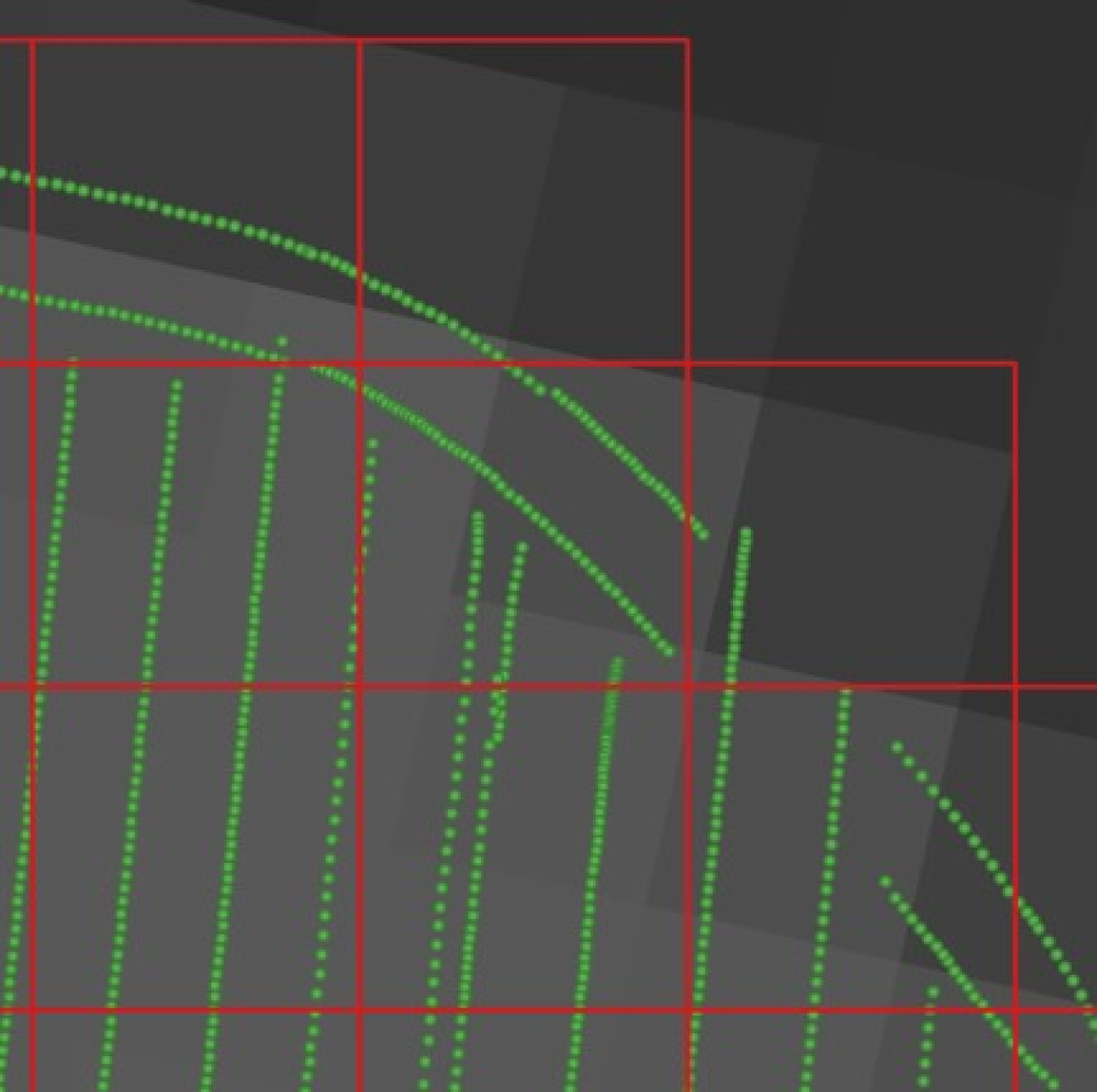
Figure 2. Crop AIQ interprets data from remote sensing. Image courtesy of INL
The Status Quo Before Crop AIQ
Previously, farmers had three alternative ways of generating the information provided by Crop AIQ. Each method had its own drawbacks, which Crop AIQ can remedy.
- Current GPS systems: Modern harvesters have GPS systems and sensors to monitor yield by measuring mass flow and grain moisture. An onboard computer captures the data, but the sensors must be calibrated every few days. That calibration can take up to half a day, which is not feasible during harvest when farmers are in a race with nature. If the calibration is skipped, the resulting yield data can be spotty and inaccurate. Crop AIQ works around this by training artificial neural networks to predict yield based on remote sensing, negating the need for a harvest monitor on every field.
- Reliance on remote sensing data: Remote sensing data often takes the form of a vegetation health index, which is derived from satellite-based spectral data in the visible near infrared range and SWIR. Because the scale is arbitrary, it does not offer a reliable way to quantify impact on production. Crop AIQ interprets the remote sensing data to generate a quantifiable and industrially relevant value measured in yield units, such as bushels per acre.
- Use of the Soil Survey Geographic (SSURGO) database: This database contains information collected by the U.S. Department of Agriculture's National Cooperative Soil Survey. The National Commodity Crop Productivity Index offers an extensive dataset, but the measurements derived from the SSURGO database have issues related to crop yield. Estimates of soil productivity are largely fixed and do not capture changes over time, and spatial resolution is limited. Crop AIQ incorporates real-time electromagnetic reflectance data collected at high spatial and temporal resolutions, delivering improved spatiotemporal capabilities.
Crop AIQ Today and Tomorrow
Crop AIQs use data from harvest yield monitors to train its artificial neural networks. A larger training set makes for a more robust model. Ideally, given the large number of current yield monitors available today, datasets could be identified and used to support regional grower groups.
A 2017 paper published in the journal BioEnergy Research details a study conducted in four counties which found that farmers could significantly increase their annual biomass production—from nearly double to 21 times more—by planting energy crops on unprofitable land. To fully achieve the gains highlighted in the study, farmers would need to adopt precision agriculture made possible by Crop AIQ.
Dr. Lynn Wendt
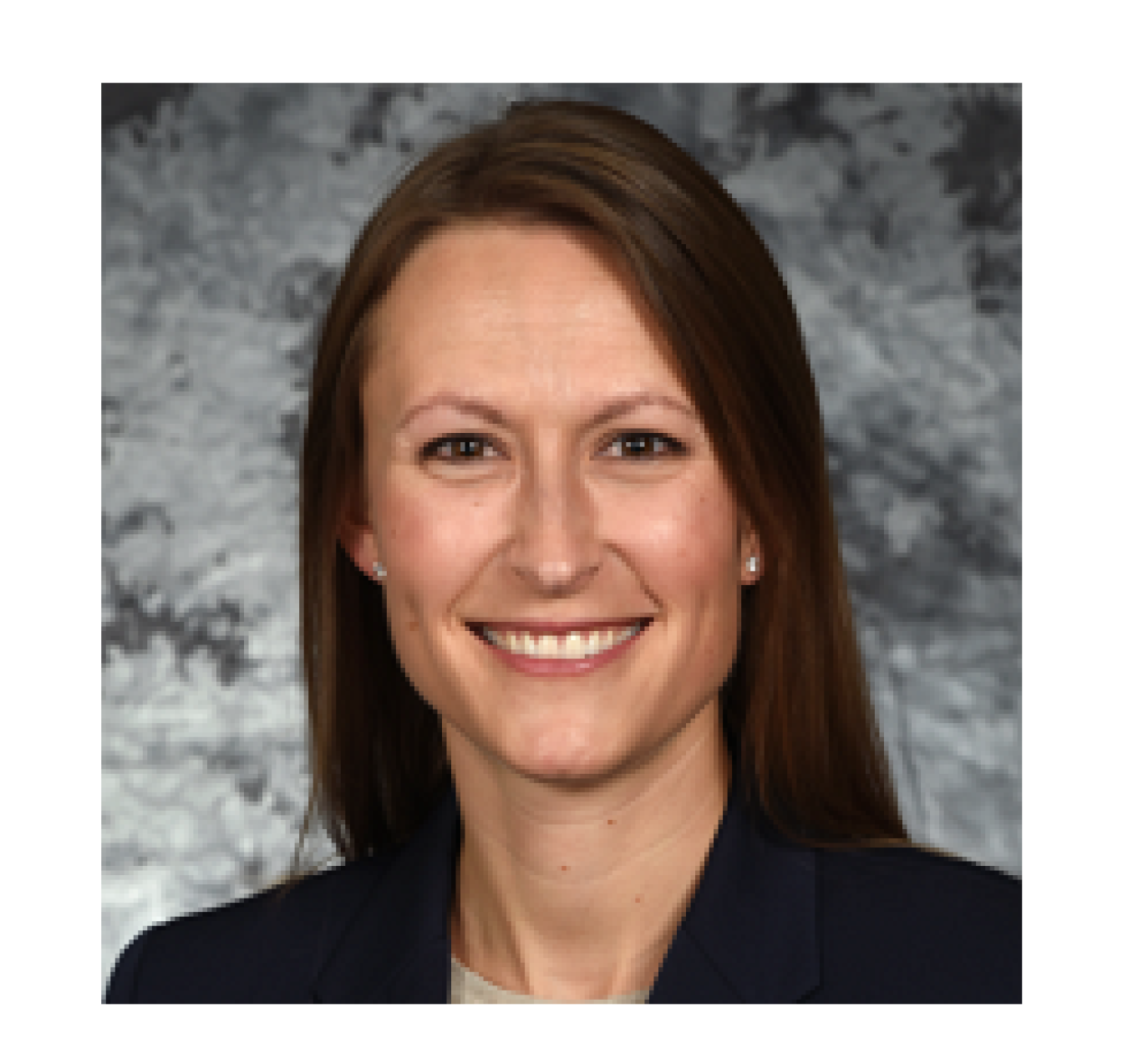
Dr. Lynn Wendt serves as the Laboratory Relationship Manager to the U.S. Department of Energy’s Bioenergy Technologies Office (BETO). Dr. Wendt is also a senior research scientist in the Energy and Environment Science and Technology Directorate at Idaho National Laboratory (INL). She has served as a principal investigator for multiple projects sponsored by BETO and has contributed to strategic planning for INL’s Biomass Feedstock National User Facility and associated programs. Dr. Wendt holds a bachelor’s degree in biochemistry from the University of Minnesota, a master's in biology from Idaho State University, and a Ph.D. in environmental science from the University of Idaho. Dr. Wendt is a bioenergy research expert in post-harvest physiology and chemistry of biomass storage systems and microbial systems for bioenergy feedstock supply chain processes. She has pioneered the development of biomass and algae material storage and handling systems that stabilize biomass materials while increasing biomass value during short- and long-term storage. Her 25 biomass-related publications and 3 patent applications are the authoritative reference on value-add biomass storage systems.